Myriad (Tulips), 2018
C-type digital prints with handwritten annotations, magnetic paint, magnets
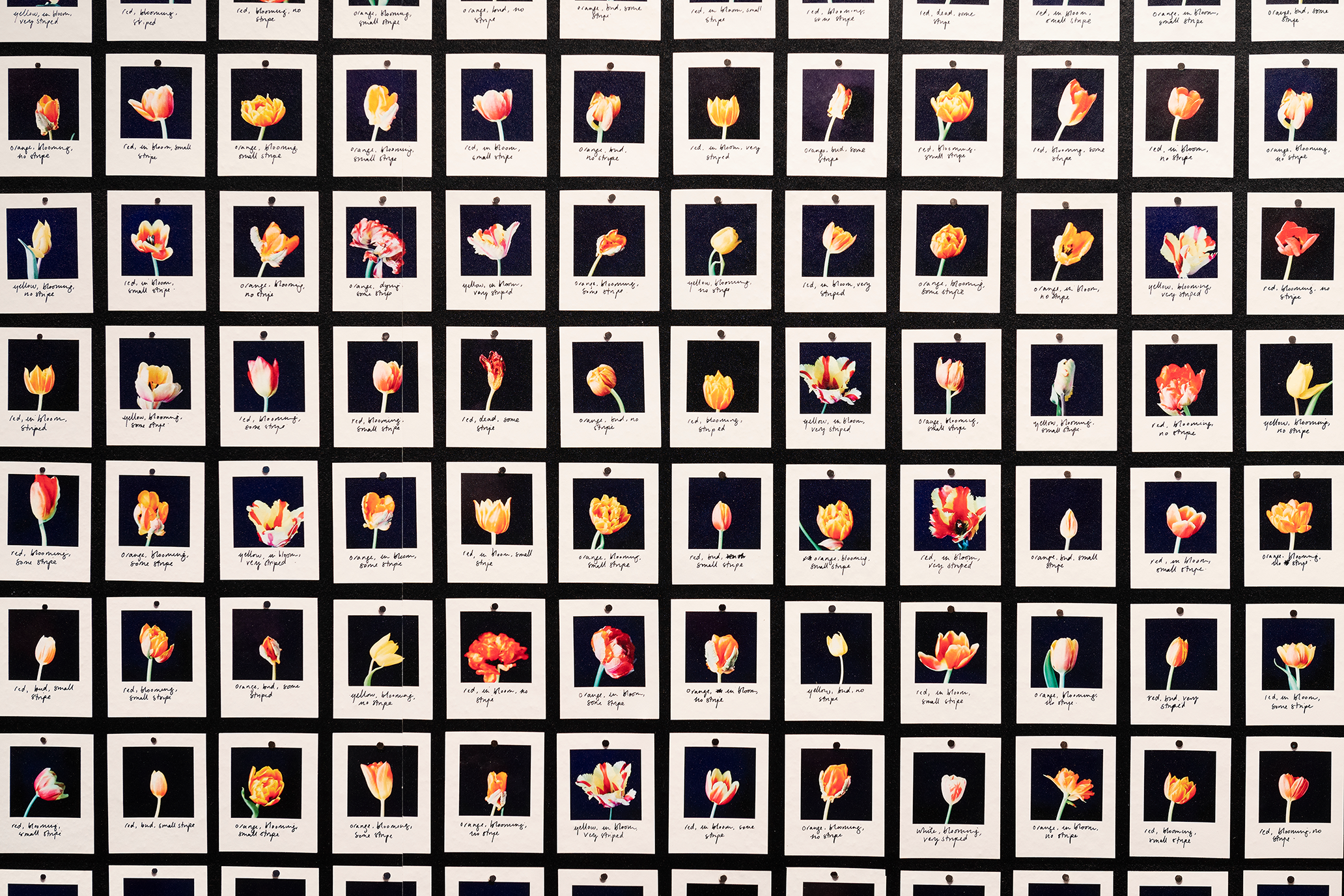
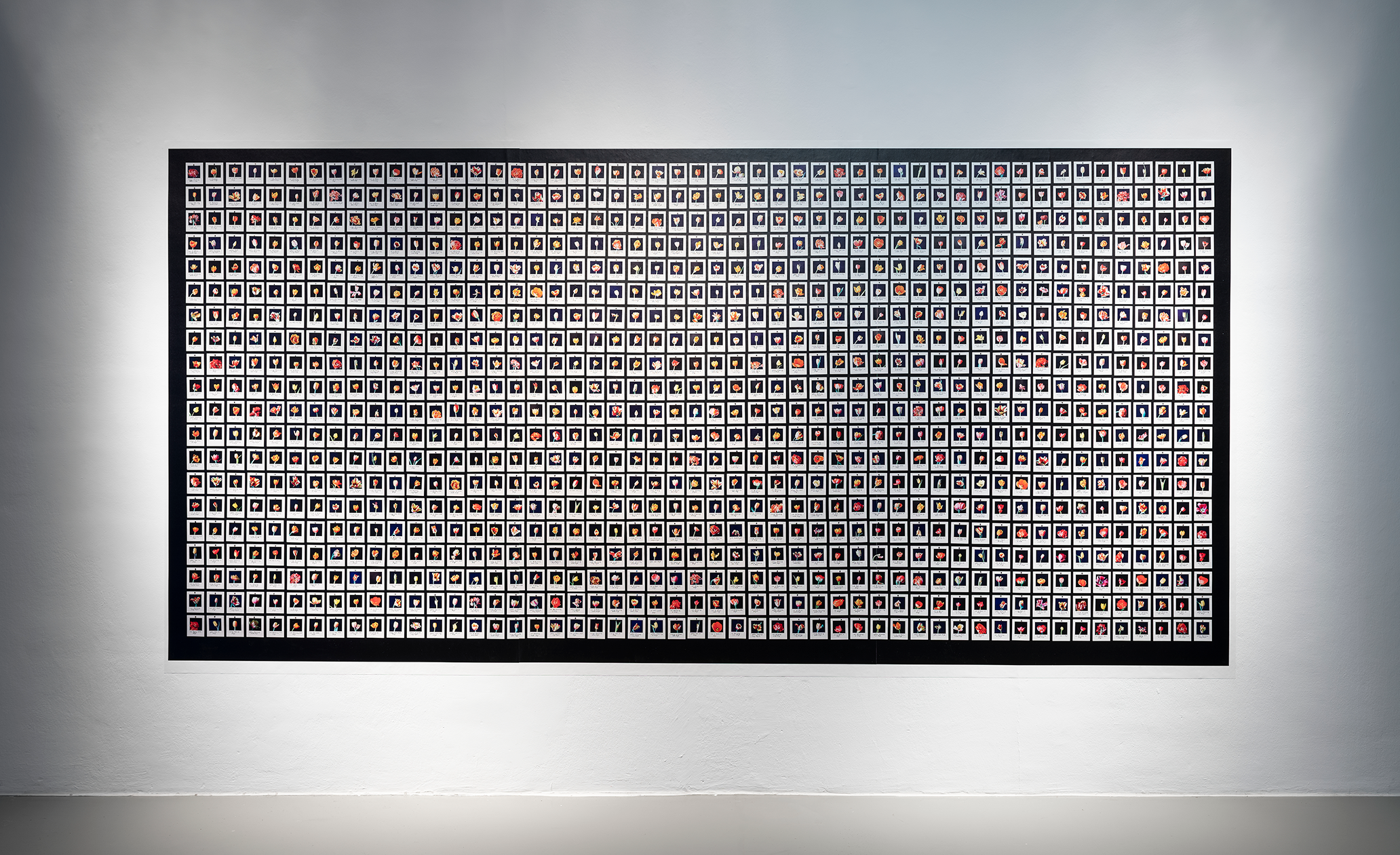
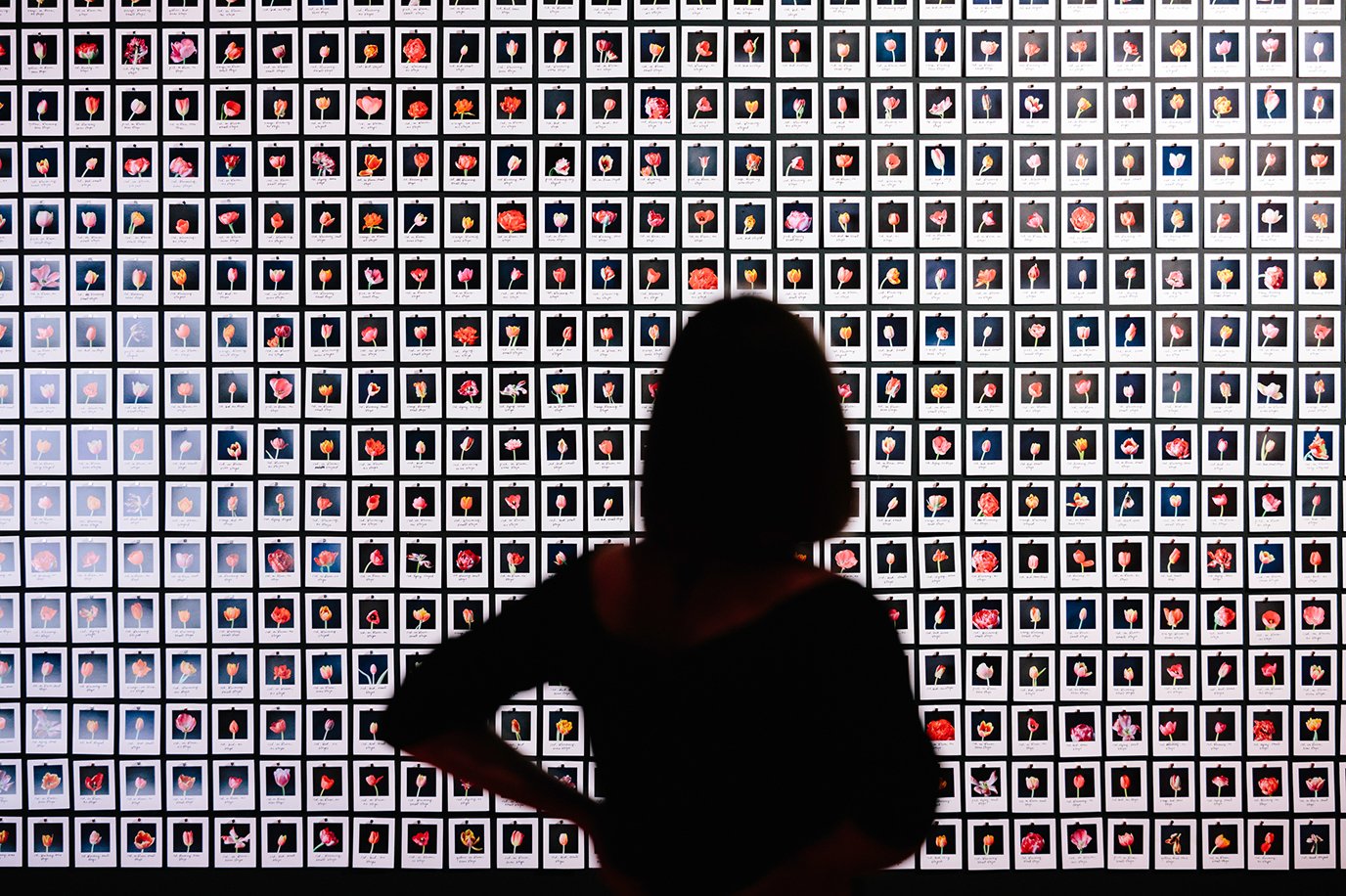
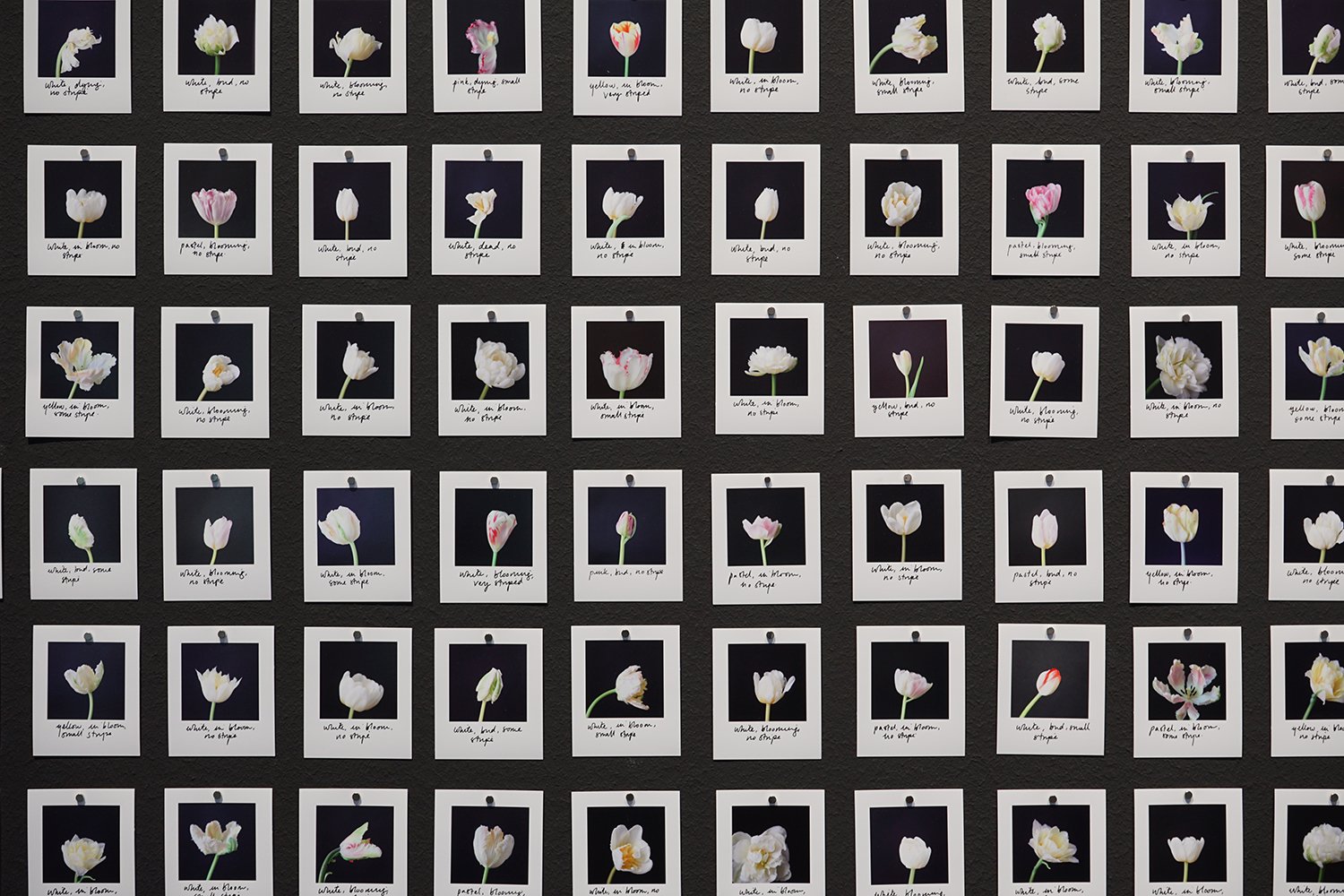
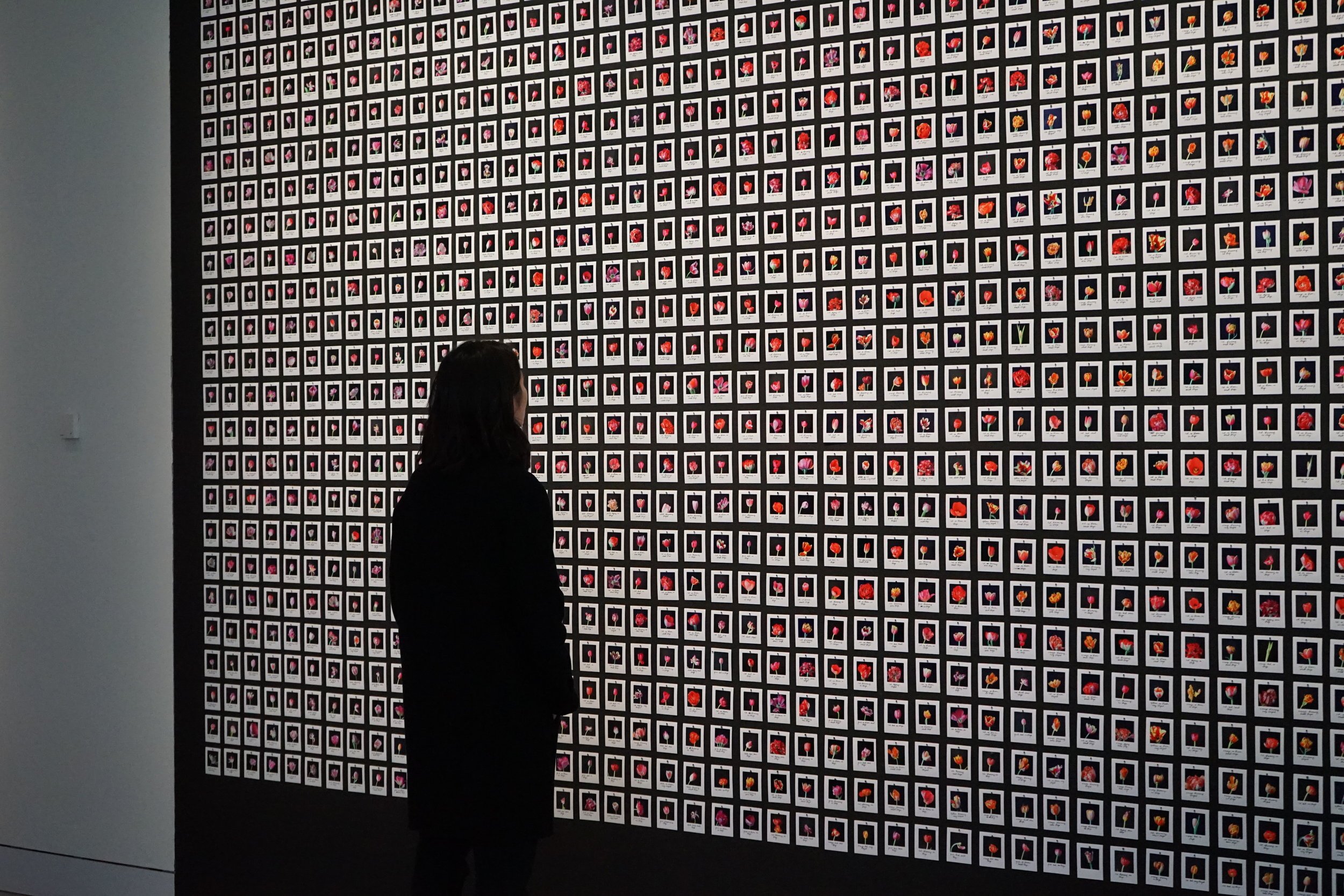
Myriad (Tulips) is an installation of thousands of hand-labeled photographs of tulips; these photographs were later used as the dataset for Mosaic Virus 2018 and Mosaic Virus 2019. By choosing to make the dataset an artwork it draws attention to the skill, labour and time that goes into constructing it, whilst also helping to expose the human element in machine learning, usually hidden by algorithmic processes.
Research and Process
Each piece of technology has its own associations and connotations: Myriad (Tulips) is surfacing some of these. I took ten thousand, or myriad of photographs of tulips over the course of three months whilst on residency in the Netherlands. The subject matter of tulips was deliberate, allowing me to make connections between speculation and value through tulipmania in the subsequent Mosaic Virus pieces but also drawing in the history of flowers in machine learning datasets. The iris flower dataset, created by British statistician Ronald Fisher, contains 50 samples of 3 different irises and is used as a test case for many statistical classification techniques in machine learning. It is included in the package Scikit-learn so that every machine learning programme that uses this package also contains within it somewhere a hidden flower dataset. This unexpected link brings the installation into the history of machine learning. But by referencing Fisher, I am also referencing the fact that he was also heavily involved in racism and eugenics (foreshadowing perhaps some of the inherent problems with machine learning, bias and datasets). Even something as simple as a flower contains within it hidden layers and narratives.
By creating my own dataset, it forces me to examine each tulip and subsequent image, and inverts the usual process of creating this type of large dataset, which are usually built using mechanical turks and imagery that has been scrapped from the internet. The project was driven by the rhythms of nature, the collection of tulips stopping not because a certain number had been collected but because tulip season had ended. The process of making datasets is almost like craft - repetitive, time-consuming, often unauthored, but necessary in order to produce something beautiful. And there is a skill to it (something that is recognised by copyright law). If the dataset is too big, if there are too many images, the results will be too good and the quirks and oddities that make it an interesting medium to explore will disappear; if it is too small it will not have enough information and become flummoxed, either producing nothing or one or two variations from the training set again and again. Therefore each photograph is carefully selected, as part of an iterative process, to produce the type of result that I desired. This is in direct opposition to a dataset such as ImageNet (a canonical dataset often used in computer vision), where there are only around 1,000 tulips out of its fourteen million images, all which have been anonymously found, categorized and labeled. I had a direct connection to the objects I was documenting. It is easy to forget in the digital age that information is physical and that things that are seen on a screen once started out in the real world. The process was physical - buying, moving, stripping hundreds and hundreds of flowers - labour that is often obscured, even in this rendering of a dataset.
The labour that is visible is that of categorisation. Computability is often accomplished by categorisation - in order for a particular image to be retrieved, or understood within a dataset it needs to have a label. In the installation each photograph has a handwritten label underneath it with some of the categories that were used - the colour of a tulip, the state of the flower, the stripiness of the petal - drawing attention to the subjectivity that accompanies such decisions. Even something as simple as a tulip is difficult to put into discrete categories (is it white or pale pink? Is it orange or yellow? Is it a bud or has it just started to bloom?). To take just one of these - colour (which has its own acknowledged difficulties in the history in the botanical classification, openly opposed by Carl Linnaeus as “a necessary or desired trait” in describing plants) - is to start to explore the haziness around the definitions and perceptions of it. Something is always lost when material form is translated into language, and the result is always a result of the person choosing words. Because machine learning is so heavily dependent on language and categorisation, there is always a human decision somewhere along the chain of using machine learning and that it is not this absolute correct thing. What I perceive as an orange flower could very well be seen as yellow to someone else. Herein lies the issue concerning datasets: implicit bias becomes virtually unavoidable. However, in using photographs that I have taken I am able to impose greater control over the meaning of the images and how they eventually are processed by the algorithm and the resulting errors or assumptions that are made are mine and mine alone.
By choosing to create a physical installation of the dataset as Myriad (Tulips), aspects about the data can be comprehended in a way that they might not be otherwise. The installation of all of the photographs is over 50 square metres, giving an overwhelming sense of the time, money and effort that goes into constructing a dataset. Each photograph is carefully affixed one by one with magnets to a specially painted black wall in a laborious process to form a seemingly precise grid. Up close, however, slants and errors come into view, evoking the imperfect and arduous human labor behind machine learning and also its imperfection.
“[Tulip Mania meant that] the order of the stock market was introduced into the order of nature. The tulip began to lose the properties and charms of a flower: it grew pale, lost its colours and shapes, became an abstraction, a name, a symbol interchangeable with a certain amount of money.” - Zbigniew Herbert
"The Abstraction of Nature", the title of a recent solo show, references the above ideas around value and the mania, but also how computer vision abstracts certain characteristics in order to "learn".
The iris flower data set is a multivariate dataset created by statistician Ronald Fisher in his 1936 paper The Use of Multiple Measurements in Taxonomic Problems. It contains the measurements of 50 specimens from 3 different types of Iris and is included in the R base and SciKitLearn so it is automatically included whenever a user downloads these programmes. Myriad (Tulips) references this history of datasets and how so many pieces of machine learning code also import a floral dataset.